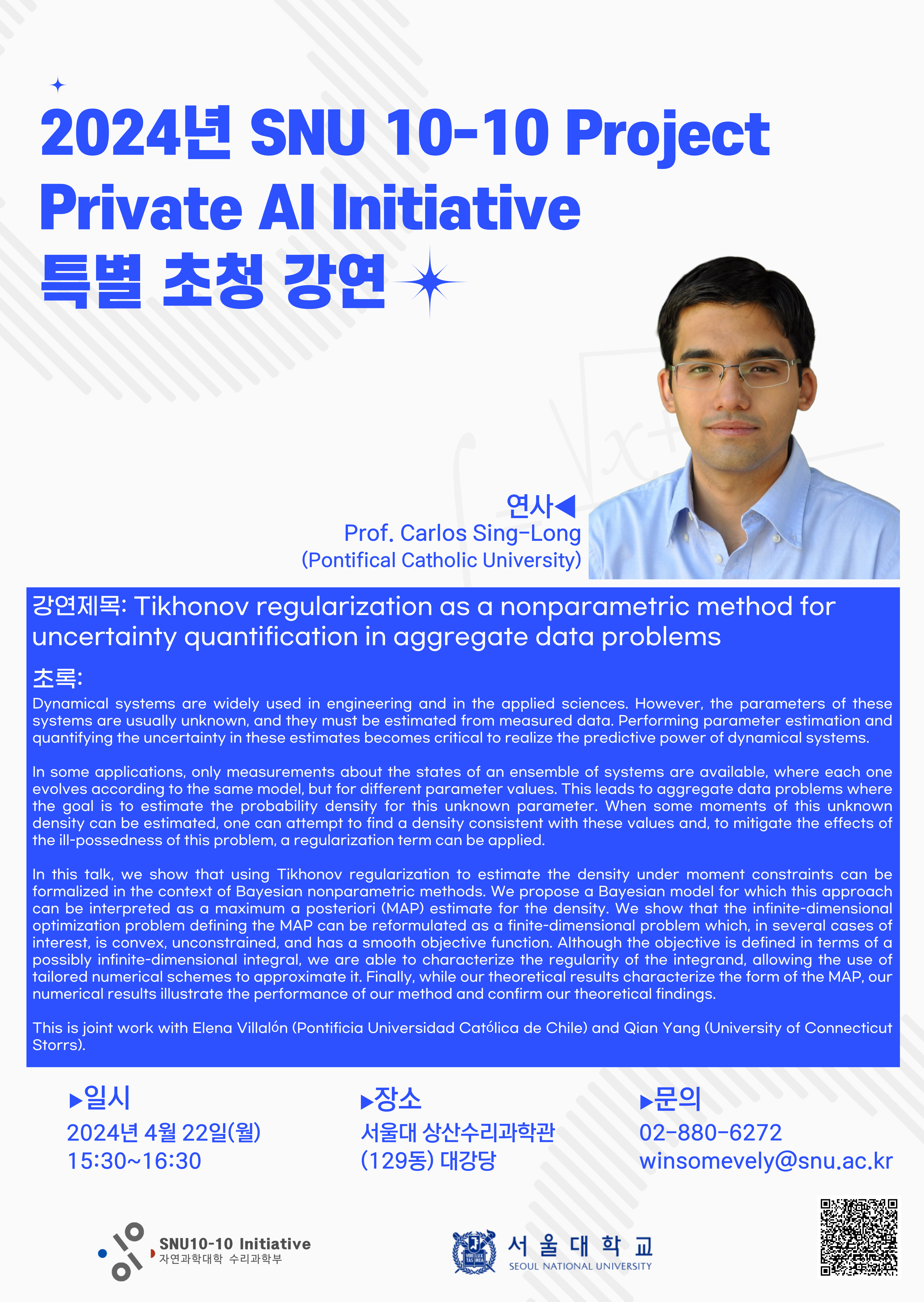
□ 장소: 서울대학교 상산수리관 대강당
□ 연사: Prof. Carlos Sing-Long (Pontifical Catholic University)
□ 강연정보
In some applications, only measurements about the states of an ensemble of systems are available, where each one evolves according to the same model, but for different parameter values. This leads to aggregate data problems where the goal is to estimate the probability density for this unknown parameter. When some moments of this unknown density can be estimated, one can attempt to find a density consistent with these values and, to mitigate the effects of the ill-possedness of this problem, a regularization term can be applied.
In this talk, we show that using Tikhonov regularization to estimate the density under moment constraints can be formalized in the context of Bayesian nonparametric methods. We propose a Bayesian model for which this approach can be interpreted as a maximum a posteriori (MAP) estimate for the density. We show that the infinite-dimensional optimization problem defining the MAP can be reformulated as a finite-dimensional problem which, in several cases of interest, is convex, unconstrained, and has a smooth objective function. Although the objective is defined in terms of a possibly infinite-dimensional integral, we are able to characterize the regularity of the integrand, allowing the use of tailored numerical schemes to approximate it. Finally, while our theoretical results characterize the form of the MAP, our numerical results illustrate the performance of our method and confirm our theoretical findings.
This is joint work with Elena Villalón (Pontificia Universidad Católica de Chile) and Qian Yang (University of Connecticut Storrs).